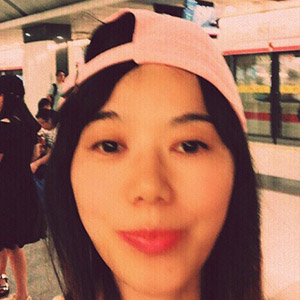
Xin Yang is a research data scientist currently based in Shanghai. With an educational background spanning three different European countries since undergraduate, she brings a unique global perspective and a diverse skill set that enriches her approach to her current role. She works in the realm of providing AI-driven marketing optimization solutions for global enterprises.
Her doctoral thesis that she will be defending in September 2023 explores solutions for optimizing targeting selection in direct mail marketing campaigns, under the supervision of Professor Kiane Goudarzi, IAE Aix.
Thesis Direction
Pr Kiane Goudarzi
Thesis Title
An empirical study on optimizing direct mail target selection
Abstract
As the oldest format of direct marketing, direct mail distinguishes itself as a unique business philosophy primarily due to its tangibility. It is costly, but with the right targeting and curated content, it can be extremely rewarding. This dissertation presents a comprehensive decision theory framework for a cosmetic firm. The firm’s ultimate goal is to optimally target an upcoming direct mail campaign, with nearly 500,000 potential customers within the Chinese market to consider. Direct access to the firm’s structural database, which covers a timespan of two years of point-of-sale micro-level data, enables us to conduct a series of analytic models and drawsubstantial conclusions. First, we measure howa customer’s past purchase behavior influences her near-future repurchase decisions. Second,we identify to what extent a firm’s direct mail campaign can spur a customer’s positive response, causally via a quasi-experimental setting. Third,we verify howmachine learning algorithms aid the decision-making process for direct mail marketing by determining the optimal targeting policy when coupled with conventional econometric methods. Finally, we evaluate whether it is economically viable to invest effort into engaging difficult customers. Patterns in our data align with previous literature. We concur that utilizing a customer’s purchase history data, rather than their demographic information, could suffice to improve the firm’s profitability. This is mainly due to the presence of persistent customer inertia (Heckman, 1981). Additionally, we also found that an imageenhancing direct mail piece could have a significant positive impact on a customer’s decision to repurchase. The theoretical threshold mechanism we adopted from Bult and Wansbeek (1995) plays a constructive role in target optimization. It allows us to dynamically account for the firm’s costs and returns with rigour. This also serves as a rare example in previous literature.
However, its effectiveness relies on the quality of the prediction of customers’ repurchase probabilities being optimal. Overall, these findings have significant implications for the role of targeting, specifically target selection, in the performance of a direct mail campaign. We view our approach to navigate this task as a quintessential representation of bridging the gap between machine learning models and econometric models within the context of managerial direct mail marketing discourse. Finally, our results only apply to single-period short-term (3 months) campaigns. The positive externality might carry over longer than 3 months, which remains unexplored.