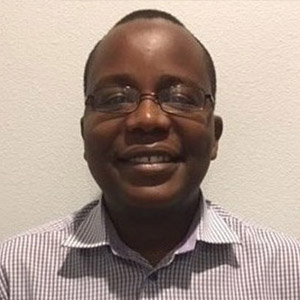
Masialeti Masialeti is an Information Systems practitioner and part-time academic with more than 14 years of experience in the Mining, Education, and IT Consulting Sectors. He has worked in both Africa and North America, and is currently IT Service Delivery Manager with Nevada Gold Mines based in the United States of America. He previously served as Technology Systems Leader, Global IT Service Delivery Manager, and IT Superintendent with Barrick Gold Corporation. Masialeti Masialeti’s research interests are Business Analytics and the application of Information systems theory to the successful implementation of digital transformation technologies such as IoT, Mobile Applications, Big Data Analytics, Machine learning, etc in the Mining industry.
In September 2021, Masialeti Masialeti defended his Executive Doctorate in Business Administration (Executive DBA) on the topic of ‘An empirical study of short interval control tasks and mobile technology fitness in an underground mine’, under the supervision of Professor Jean-Fabrice Lebraty, Jean Moulin Lyon 3 University.
Thesis Direction
Pr Jean-Fabrice Lebraty
Thesis Title
An Empirical Study of Short Interval Control Tasks and Mobile Technology Fitness in an Underground Gold Mine
Abstract
Digital transformation has become a key strategic objective for many mining companies. Mobile technology has recently become a platform of choice for the digital implementation of short interval control (SIC) tasks. This study empirically investigated the SIC tasks of planning, execution, and control via mobile technology in the mining industry at Turquoise Ridge Underground (TRU) gold mine in Nevada, the USA. Specifically, the proposed new task technology fit (TTF) theory by Howard and Rose (2019) was used as a framework of analysis to assess the fit between SIC tasks and mobile technologies to drive miners’ performance at TRU. A web survey was carried out among 200 sampled underground miners. The resulting research model was analysed using the partial least squares-structural equation modeling (PLS-SEM) approach. The research findings reveal that the interactive effect of SIC task characteristics and mobile technology characteristics mediated by TTF has no statistically significant effect on underground miner reactions, utilisation of mobile technology, and performance outcomes of miners. However, the direct effect of mobile technology characteristics on TTF, TTM – “Too Much” and TTM – “Too Little” is statistically significant. These results revealed that TTF significantly predicts underground miner reaction, utilisation, and performance outcomes. The study suggests that Task-Technology Fit (TTF) results in better prediction, thus allowing for better decision making around improving the SIC process, which results in better performance of underground miners. However, Task-Technology Misfit (TTM) is unpredictable and therefore makes it challenging to make decisions on improvement, thus negatively impacting performance. In addition, the results suggest that we can assess the differences between TTF and TTM in terms of the capability of performance prediction. Theoretical and managerial implications are suggested because of the findings of the study.